Generative AI Use Cases in Healthcare: How the Tech Is Transforming the Industry
What’s going on in healthcare today? Fewer clinicians, higher costs, and more admin work are slowing down care and burning out teams.
Gen AI promises a solution — faster workflows, fewer manual tasks, more time for patients. But many generative AI use cases in healthcare are still stuck in pilot mode. And with big questions around safety, regulation, and data privacy, many organizations aren’t sure how to move forward.
This guide is here to help. We highlight applications of generative AI in healthcare in medical software development services: what’s actually working today, where early wins are showing up, and how to safely explore Gen AI without compromising patient care or compliance.
Generative AI, and namely large language models (LLMs) like GPT, are a new kind of AI that can create content — not just sort or label it. Instead of being fed rules or structured data, these models learn from huge amounts of text and examples. They spot patterns, then use those patterns to generate new outputs.
In healthcare, that means things like writing a clinical summary, drafting a referral, or auto-filling a pre-auth form — all in seconds.
What makes LLMs different from older AI?
Traditional methods in healthcare mostly worked with structured data — lab values, medication codes, EHR fields.
LLMs use messy, unstructured data: notes, transcripts, PDFs, even conversations. And this is crucial for healthcare, where most data is unstructured.
Used safely, they’re like a medical intern that never sleeps, can summarize an entire EHR in seconds, and speaks your tone.
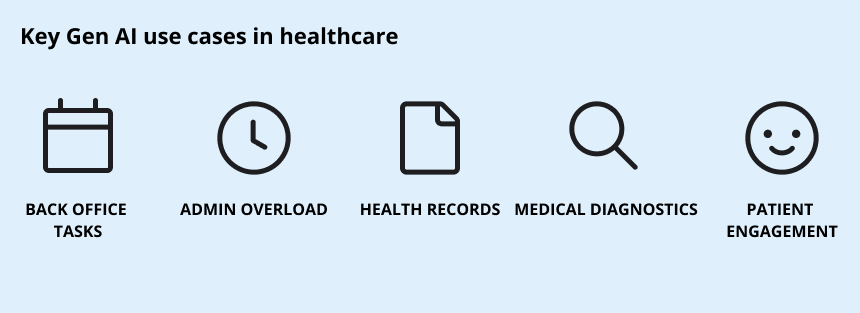
Back office tasks and administrative overload
Admin work in healthcare has always felt out of control.
We’re talking about everything from scheduling and referrals to insurance claims, patient records, and chasing down authorizations. It’s a lot. And most of it still falls on already-overworked staff and clinicians.
According to McKinsey, the U.S. spends over $1 trillion a year on healthcare admin. 90% of related calls are still done by humans. That’s a massive cost — in time, money, and focus.
And with the ongoing staffing shortage (a 100,000-worker gap expected by 2028), this isn’t sustainable. Doctors are spending 28 hours a week on admin. Claims and office staff — 34 to 36 hours. That’s time not spent with patients.
This is exactly where generative AI can step in and make a real difference.
Here’s what that looks like in practice today:
- VoiceCare AI is piloting its voice assistant, Joy, with Mayo Clinic. Joy handles common tasks like benefit checks and prior auths — and sounds like a real team member. It shortens staff wait times and frees up time by replacing hold music and manual data entry with fully automated, documented calls.
- Innovaccer’s Agents of Care™ work like digital teammates. They take on everyday tasks — scheduling, coding, answering patient questions — across 80+ EHRs. That means less time on copy-paste work and more time for real care. Plus, they plug into existing systems, so there’s no need for big changes or heavy lifts.
- Cognizant and Google Cloud have teamed up to create AI tools that automate some of healthcare’s most repetitive and costly tasks. These include call center operations, handling appeals and grievances, provider management, and contract processing. The result: reduced manual work, faster processes, and more efficient teams.
If you’re building a digital health product or modernizing a provider organization, admin workflows are an ideal starting point for generative AI in healthcare. They’re easier to automate than other workflows and have a big impact. The ROI shows up quickly — saving time, improving operations, and making staff happier.
Thinking about using Gen AI in the back office?
At Digiteum, we help teams move from strategy to delivery — starting with admin automation and scaling to broader transformation.
Contact usHealth records
Clinicians are also overloaded with documentation. They spend hours every day writing notes, updating EHRs, filling out referrals, and coding for billing. It’s repetitive, high-pressure work that takes time away from patient care.
No surprise that 8 in 10 US doctors list paperwork as a top cause of burnout — often adding little value to actual outcomes.
This is where Gen AI is already delivering real results. Several use cases of generative AI in healthcare show that LLM-powered tools can listen to visits (with consent), understand the conversation, and create clean, structured notes in real time.
A good place to start? Note automation in high-volume areas like primary care, urgent care, or emergency medicine — where speed and accuracy matter most.
- Kaiser Permanente rolled out an AI scribe across 600+ clinics and 40 hospitals. It’s been used in over 4 million visits. Physicians report less time at the keyboard and more time with patients — with better documentation quality, too.
“For example, many physicians are now dictating aloud their results during a physical exam, whereas before they may not have said anything. To me, this is a great example of how emerging technologies can help support our care teams in delivering superior care experiences for our members and patients.” Dr. Daniel Yang, VP of AI and Emerging Tech, Kaiser Permanente.
- Augmedix Go is now used in emergency departments at HCA Healthcare. It’s built for loud, fast-paced settings, where conversations can be messy and non-linear. The tool uses a Bluetooth mic and mobile app, so clinicians stay hands-free and focused on care. It listens, understands, and generates accurate notes — even with background noise. So far, it’s earned a 99% patient consent rate and is already cutting down documentation time and stress for busy ED teams.
Medical diagnostics
Healthcare is generating more diagnostic data than ever — from imaging and surgical video to continuous monitoring. But nearly 97% of medical data goes unused, and 80% of it is unstructured — buried in formats like free-text notes, scans, and videos.
This gap slows decisions and burdens clinicians with manual review.
Luckily, AI models like LLMs and generative models can quickly process unstructured data, turning complex information into clear, actionable insights. And many Gen AI use cases in healthcare prove that.
In medical imaging analysis, AI is making a difference by reducing scan noise, speeding up image acquisition, and spotting abnormalities.
- GE HealthCare is teaming up with AWS to bring Gen AI into diagnostics. Their tools are already helping reduce scan noise, shorten imaging times without losing detail, and automatically flag issues in MRI, CT, and other scans. As a result, users get faster results, less manual review, and clinical decision support — all without adding to the team’s workload.
- ScribblePrompt, developed by MIT, Harvard, and MGH, is a new Gen AI tool that speeds up and improves medical image segmentation. Instead of manually labeling everything, they just mark a few spots, and the AI fills in the rest. It’s already shown to cut annotation time by 28% and boost accuracy by 15%, making diagnostics faster, easier, and more consistent across imaging types.
- Quibim, a precision medicine startup, raised $50 million to create AI models that analyze MRI, CT, and PET scans to detect diseases like cancer, Alzheimer’s, and liver conditions earlier. Their platform, QP-Insights, helps predict how diseases will progress and how treatments will work, improving clinical trials and patient care. Quibim’s solutions meet regulations and are already used by hospitals and drug companies to improve treatments and develop new medicines.
Patient engagement
Patient engagement is crucial for improving care, but many healthcare systems face challenges. Hospital administrators and insurance executives know that patient portals are often underused. These platforms are too complicated, making it difficult for patients to manage their health or communicate effectively with providers.
Additionally, clinical notes are filled with medical jargon that patients don’t always understand. This can create barriers to self-reporting and symptom tracking, leading to gaps in care.
Generative AI can help solve these problems. It can simplify complex medical language, making it easier for patients to understand their care plan. It can also improve patient engagement. When it comes to how to create a patient portal that’s simple and effective, generative AI can be the solution, making it easier for patients to manage their health.
- HeyGen helps close the language gap in healthcare. Many patients struggle to understand medical instructions — especially if English isn’t their first language. Using generative AI in healthcare, HeyGen turns complex information into short, clear videos in 175+ languages, voiced by realistic, human-like avatars. In a pilot with Thai-speaking patients, the videos helped them better understand radiation treatment. This cut down confusion and saved time for care teams.
- Chronisai, a chronic care platform developed by Digiteum for a US healthtech startup, helps diabetes patients track nutrition using voice input instead of touchscreens. Powered by Generative AI, it converts voice data into structured information, which is saved in the app and shared with physicians. This makes it easier for patients to report their nutrition and for doctors to track their health.
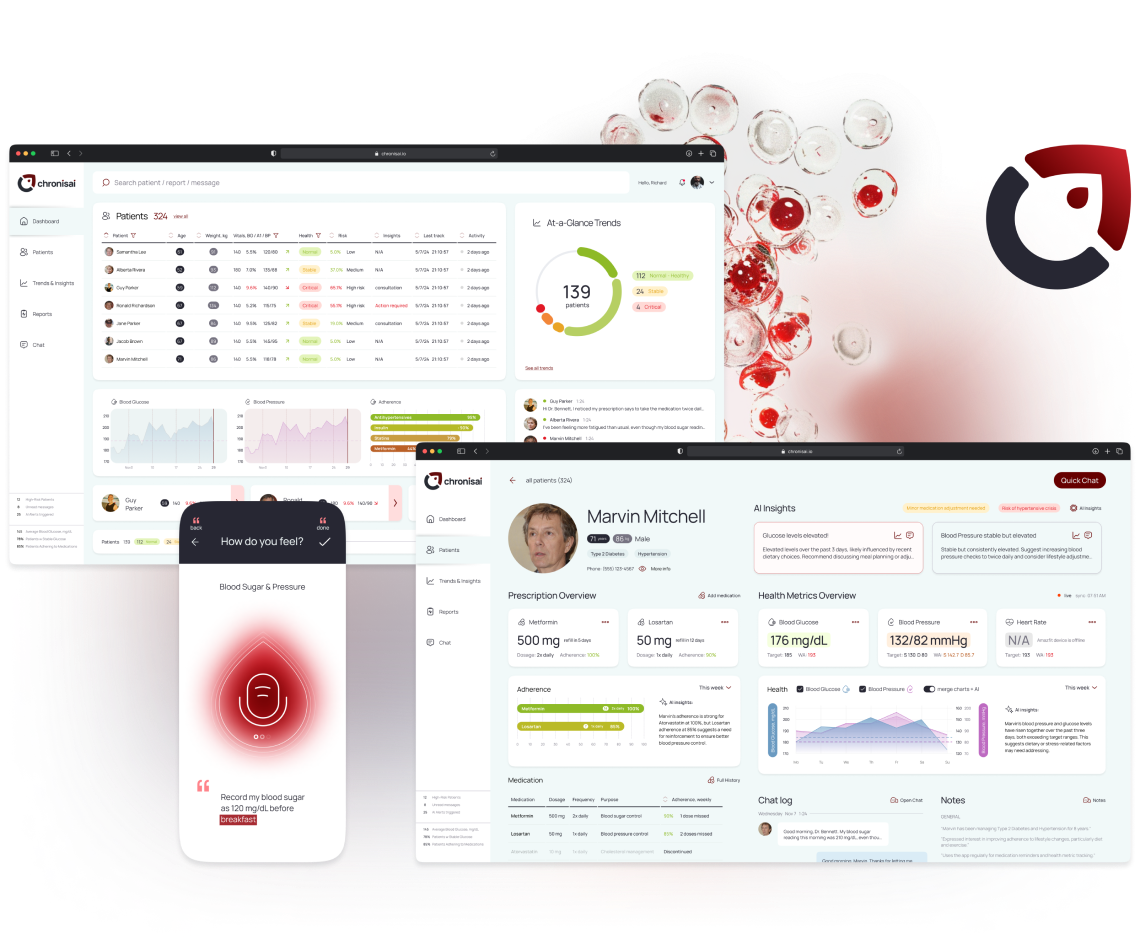
Want to improve patient engagement with Gen AI?
Digiteum will help you keep patients on track with their health and provide doctors with better data to inform decisions.
Send a requestHallucinations and accuracy risks
A major barrier to healthcare AI adoption is hallucination — when models produce confident but incorrect responses. This risk is especially critical in use cases like summarizing radiology reports or chatting with patients, where factual accuracy is essential.
“If we’re using LLMs to simplify radiology reports to make them understandable to patients or for using chat GPT or other LLMs to answer queries, answer patient queries, we need to ensure that it’s 100% correct before deploying it in real-time.” Manisha Bahl, MD, breast imaging division quality director and breast imaging division co-service chief, Massachusetts General Hospital.
Today’s LLMs lack built-in fact-checking and are prone to fabricating details, making them unreliable for unsupervised use in clinical workflows. For healthtech leaders and startup teams building with Gen AI, this means manual validation is still required, and trust from clinicians will be slow to earn until the tech improves.
Bias and fairness in training data
Bias is another challenge for generative AI in healthcare. These models learn from existing data — and that data often comes with built-in flaws. In primary care, for example, triage tools sometimes rely on patient-entered info that may not be technically wrong but still leads to misleading outputs. LLMs are no different: biased input leads to biased results.
There’s also an access gap. Patients who are more educated or tech-savvy are more likely to benefit from AI tools, while others risk being left out.
In theory, Gen AI can help detect bias, clean up data, and make healthcare more inclusive. But it won’t happen by default — it has to be intentional.
Overreliance and deskilling
Another growing concern with generative AI in healthcare is overreliance — when doctors start trusting the system more than their own judgment. The fear isn’t just about mistakes, but about what happens over time: deskilling. If clinicians lean too much on AI for diagnosis or treatment advice, they may lose confidence in their decisions or stop developing the hands-on experience that’s critical in care.
We’re already seeing this play out. In one UK study, junior doctors said they started second-guessing themselves because of constant AI alerts. Others worried that less experienced colleagues were becoming overconfident just because the AI “said so,” even when they didn’t fully understand the case.
The real risk? When the system gets it wrong — or goes offline — clinicians may not be ready to step in. That’s why it’s key to build Gen AI that supports, not replaces, clinical thinking.
Data security
In healthcare, data security is a matter of trust and compliance. Patient records contain some of the most sensitive personal information, and any exposure can cause serious harm, both to individuals and the organizations that care for them.
That’s why open-source or consumer-grade Gen AI tools can be risky. Many don’t meet the strict security and privacy standards required in clinical settings. For example, if a Gen AI tool trained on sensitive EHR data leaks information — even unintentionally — it could trigger HIPAA violations or legal consequences.
For healthtech leaders building LLM-powered tools for clinicians or patients, technical skills alone aren’t enough. What teams need is a partner who understands both worlds — someone with a strong track record in healthcare software product development and hands-on Gen AI experience.
That’s where Digiteum comes in.
- Healthcare is our core focus. We’ve worked on real-world solutions for companies like Takeda and the Lymphoma Research Foundation — from clinical trial platforms to patient engagement tools. You won’t need to onboard us on compliance, data standards, or clinical workflows — we already know how to build for this industry.
- We’ve worked across the full healthcare product lifecycle — from discovery and UX design to development and testing — and can jump in at any stage. Also, we can build an entire product from the ground up, like we did for the Gen AI-powered Chronisai chronic care app.
- We’ve helped build solutions like Chronisai (US), DXRX precision medicine platform (UK), and a personalized therapy management platfrom (US) — all compliant and used by patients and providers across the US and Europe.
- At Digiteum, we believe that any AI development — including Gen AI — starts with proper data readiness. And this is something we do exceptionally well. Our portfolio features big data projects where we turn raw data into powerful outcomes.
Let’s talk about how we can help you achieve more
Since 2010, Digiteum has been helping our clients to build reliable and compliant healthtech products. If you're looking for the same level of expertise, we’d make a great team.
Contact us